Building a Better Home Price Index
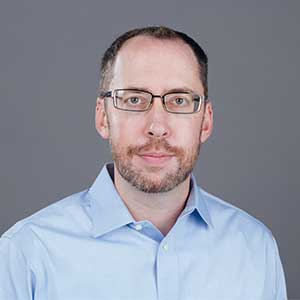
Patrick Smith
Assistant Professor, Finance
Building a Better Home Price Index
Most home price indexes do not account for home improvements which may skew results.
There are numerous economic indicators that track the health and growth of the economy and among the most important of those indicators is home price indexes (HPI). The most notable and widely used HPI is the S&P CoreLogic Case-Shiller Index (known primarily as “Case-Shiller“) which is released on the last Tuesday of each month.
The Case-Shiller HPI measures home price movements for residential real estate both at the national level and for 20 metropolitan areas within the United States by using a repeat-sales methodology that estimates price changes over time using sales of the same house. Although the Case-Shiller HPI adjusts for some changes to the quality of the house (i.e., an addition to the house), it does not account attributes such as the depreciation of unoccupied houses that have fallen into disrepair or significant home improvements (i.e., a remodeled kitchen) that could impact the sales price. The changing attributes of the house – though not reflected in the HPI - may substantially impact the index, which in turn, impacts the economic indicators.
Dr. Patrick Smith, assistant professor of finance at San Diego State University’s Fowler College of Business, and Dr. Adam Nowak, associate professor of economics at West Virginia University’s John Chambers College of Business and Economics, set out to create a more complete HPI that takes these attributes into consideration in order to form a more accurate measure of house prices.
To accomplish this goal the professors devised a system to identify keywords and phrases such as “renovated”, “granite” and “stainless” in the written descriptions of the listing agents’ postings using Multiple Listing Service (MLS) data provided by Redfin. Recognizing that the list of keywords and phrases may vary over time and across geographies, they created a machine learning* algorithm to automate the keyword selection process for the nine metropolitan areas they examined. For the sake of consistency, the algorithm used only those houses whose standard attributes (i.e., square footage, bedrooms, bathrooms) stayed constant across the repeat sales. This is similar to the methodology used by the Case-Shiller HPI.
Unlike the Case-Shiller HPI methodology, the researchers’ algorithm takes identifies
and controls for the relevant keywords and, in doing so, distinguishes which houses
increased in value simply through appreciation or through upgrades that could have
a positive impact on home values. “We found that the magnitude of the change to physical
characteristics to a house varied widely within a single zip code in those metropolitan
areas we studied,” said Smith. “Our approach allowed us to separate the price changes
based on house appreciation from those associated with the improvements or deterioration
of the house.”
After analyzing the results, the professors were able to identify and adjust for home price increases associated with renovations to the house thereby providing a more accurate measure of pure price appreciation.
“In conclusion, we found the Case-Shiller HPIs are biased because they do not properly control for improvements to the property (or the lack thereof),” noted Smith. “Secondly, our data-driven textual analysis approach allows us to identify and control for the improvements. Thus, our quality-adjusted HPIs are more accurate than the Case-Shiller HPIs.”
*Machine learning is a type of artificial intelligence (AI) where a computer uses information inherent in a data set to determine patterns and to correlate statistical information. In this case, the researchers subjected their algorithm to machine learning to determine the correlation between house prices, the sales dates, and keywords in the written descriptions about the house to indicate how various upgrades to a house impacted its sales value.
Note: This research was funded by the Fowler College of Business