Fowler Professors Secure Grant to Predict and Prevent U.S. Navy Equipment Failures
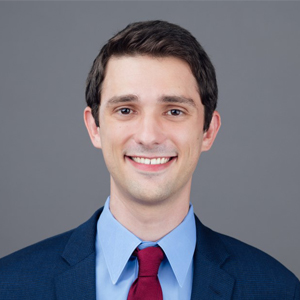
SDSU management information systems professor, David Goldberg
In fiscal year 2019, $41.4 billion was appropriated to the U.S. Navy for operations and maintenance expenses. This represents 21.3 % of the Navy’s total budget and accounts for its largest expenditure. In that same year, the Navy calculated that 48.7% of its total manpower expenses were targeted at equipment operation and maintenance.
Three management information systems professors from the Fowler College of Business at San Diego State University have proposed a data-driven solution aimed at analyzing and prioritizing needed maintenance on critical equipment. This would allow the Navy to allocate maintenance resources before the equipment failure occurs.
SDSU Professors Developing Model to Predict and Prevent Equipment Failure
The professors, principal investigator David Goldberg and co-investigators Bongsik Shin and Aaron Elkins, have been provided a grant of nearly $150,000 to develop a machine-learning text-mining model that predicts equipment with the highest risk of failure based on keywords and phrases within the Navy’s own maintenance logs. Predictors include equipment type, date of last servicing, utilization level, and equipment age.
“Our text mining approach will involve using machine learning to determine a list of ‘smoke terms’ that predict which items of equipment require maintenance,” said Goldberg. “A smoke term is a word or phrase that occurs very frequently in equipment maintenance logs that is near requiring servicing.”
Figure 1. Overview of Proposed Predictive Maintenance Architecture
Rolling Out the Process
To determine the smoke terms, the professors doing the research created an online interface that allows them to identify maintenance issues affiliated with each item (for example, they might check the box next to a phrase like “hydraulic pressure comes at the wrong level, possible blown gauge,” identifying the problematic phrase). The algorithm would then highlight specific phrases, like “blown gauge,” to identify which equipment is due for maintenance and the specifics of those maintenance issues.
This process would take approximately two years and would be rolled out in seven key phases:
- Requirements gathering — The researchers will meet with the Naval personnel at NSWC Corona to gather the data and get a full understanding of the maintenance logs in order to develop a detailed protocol for identifying maintenance needs within the logs.
- Data cleaning — Once the data is retrieved from the Naval logs, the researchers will remove extraneous white spaces, foreign characters, and typos from the text.
- Manual tagging analysis — The research team will review the cleaned data to manually tag phrases and words that indicate which equipment needs servicing (these make up the smoke terms).
- Text mining model development — An algorithm using the text mining model will be created to scan the data from smoke terms to identify and prioritize equipment in need of service.
- Integrated model development/tuning — Additional information (equipment type, last service date, equipment age, etc.) would be merged into the algorithm, and the model would be fine tuned to ensure the most accurate performance possible.
- Dashboard development – A user-friendly dashboard-style interface will be developed that allows users to sort maintenance logs in order to determine which equipment needs servicing. The smoke terms will be identified so that users can understand why certain equipment was recommended for maintenance, allowing them to allocate resources accordingly.
- Preparation of the final report — The researchers will prepare a summarization of the findings at NSWC Corona. The summary will be delivered with an oral presentation to resolve any questions or concerns.
Student Involvement
While this project was developed to help the Navy pinpoint and remedy equipment maintenance issues, it also contributes greatly to the field of research in the area of text mining and offers students the opportunity to engage in a real-world educational experience. “Student assistants will provide invaluable technical assistance while getting project-oriented experiences beyond their classroom assignments,” said Goldberg. “The faculty researchers working on this project will provide them with the expertise and training needed to assure the project proceeds on an orderly timeline and meets major milestones.”
Once the algorithm is put into place and perfected, Goldberg is hopeful that the Navy can eventually use this system on a wider scale. “We’ll start with a proof-of-concept to make sure that the tools are working as well as possible before we upgrade the system for enterprise-wide use,” he said. “If that happens, this system could be instrumental in allowing the Navy to monitor and remedy potential problems before they become critical.”
Note: This research was funded by the Naval Engineering Education Consortium (NEEC)